Knowledge Bases
A knowledge base is a computer-processable collection of knowledge about the world. We construct and mine such knowledge bases.
Graph Mining
Graphs are a near-universal way to represent data. We are concerned with mining graphs for patterns and properties. Our particular focus is on the scalability of such approaches.
scikit-network: scikit-network is a Python package for the analysis of large graphs (clustering, embedding, classification, ranking).
Social Web
The Web has evolved more and more into a social Web: content is produced and shared by users. In the DIG team, we follow and anticipate developments in this area.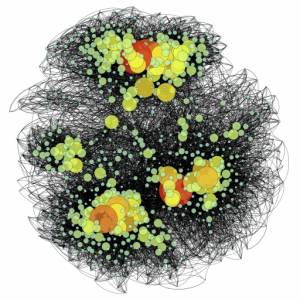
- Community detection: We are investigating means to detect and distinguish social communities on the Web.
- Social Relations: We investigate the optimal investment in social relations from a theoretical point of view.
Language and Relevance
Computer science is not just about computers. In this area of research, we investigate how humans reason, and what this implies for machines.
- Simplicity Theory:
Simplicity theory seeks to explain the relevance of situations or events to human minds. See http://www.simplicitytheory.science
- Relevance in natural language: The point is to retro-engineer methods to achieve meaningful and relevant speech from our understanding of human performance. Read this paper. Read more on this.
- Communication as social signalling: We apply game theory and social simulation to explore conditions in which providing valuable (i.e. relevant) information is a profitable strategy. Read this paper. Read more on this.
Machine Learning for Data Streams
We investigate how to do machine learning in real time, contributing to new open source tools: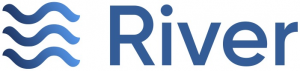
- River: a Python library for online Machine Learning
- MOA: Massive Online Analytics, a framework for mining data streams (in Java)
- Apache SAMOA: Scalable Advanced Massive Online Analytics, an open source framework for data stream mining on the Hadoop Ecosystem